Dagstuhl Seminar 20452
Decision-Making Modeling and Solutions for Smart Semiconductor Manufacturing
( Nov 01 – Nov 05, 2020 )
Permalink
Organizers
- Chen-Fu Chien (National Tsing Hua University - Hsinchu, TW)
- Hans Ehm (Infineon Technologies - München, DE)
- John Fowler (Arizona State University - Tempe, US)
- Lars Mönch (FernUniversität in Hagen, DE)
Contact
- Andreas Dolzmann (for scientific matters)
- Susanne Bach-Bernhard (for administrative matters)
Impacts
- Identifying potentials and impacts of lead-time based pricing in semiconductor supply chains with discrete-event simulation : article in WSC '21: Proceedings of the Winter Simulation Conference - Welling, Tobias Leander; Quintao Noel, Ludmila; Ismail, Abdelgafar - New York : ACM, 2021. - Pages 1–12.
- Simulating and evaluating supply chain disruptions along an end-to-end semiconductor automotive supply chain . article in WSC '21: Proceedings of the Winter Simulation Conference - Jaenichen, Friedrich-Maximilian; Ismail, Abdelgafar; Martens, Christian James; Dörrsam, Volker; Ehm, Hans - Los Alamitos : IEEE, 2021. - pp. 1-12.
Schedule
The Industry 4.0 vision is a frequently discussed topic in manufacturing enterprises in Europe, Asia, and North America. It is expected that advanced technologies such as Cyber-Physical Systems, Internet of Things, cloud computing, and big data technologies enable the emer¬gence of smart manufacturing systems. A smart factory promises to bring trans¬parency to manufacturing facilities by integrating technological advances in computer networks, data integration, and analytics. At the same time, critical questions are asked related to the benefits of Industry 4.0. It is mainly criticized that the requirements and consequences of Industry 4.0 regarding future production planning and control strategies are not fully understood or not even taken into account in the overall Industry 4.0 conception, i.e., many of key decision processes are not included.
The semiconductor industry is capital intensive. The manufacturing process is very complex due to reentrant flows in combination with very long cycle times and multiple sources of uncertainty. This industry is an extreme field for production planning and control solutions from algorithmic point of view, as well as from a software and information systems point of view. The degree of automation was always and is still high compared to other industries. On the one hand, one can argue that in wafer fabs elements of smart manufacturing are already realized, namely most of manufacturing information is available in real-time, the manufacturing process is paperless, lots can be uniquely identified and located, and collaborative human-machine interaction exists. On the other hand, there are significant differences in automation efforts related to manual work-intensive industries such as automotive or air-craft manufacturing where assembly operations are performed in flow lines. In addition to shop-floor control concerns, supply chain management problems have become more and more important which necessitate a horizontal integration of the semiconductor supply chain and digital transformation for the industry ecosystem.
The major objective of the proposed Dagstuhl Seminar is related to developing a research agenda for making smart semiconductor manufacturing decisions and the information systems to empower flexible decisions for smart production. The research agenda will be developed around the following two main topics:
- Topic 1: Novel decision-making approaches that exploit the huge amount of available data and orchestrate the interrelated decisions
- Topic 2: Future information systems for decision support and facilitating digital transformation
This includes innovative modeling approaches for supply chain planning and more detailed production planning and scheduling in semiconductor manufacturing and an analysis of requirements for next-generation information systems that support such decisions.
One of the expected outcomes of the seminar consists of developing a significant draft of a concept for a simulation testbed which allows for assessing smart planning and control decisions in the semiconductor industry. The purpose of this seminar is to bring together researchers from different disciplines including information systems, computer science, industrial engineering, supply chain management, data science, and operations research whose central interest is in decision-making for smart semiconductor manufacturing. Moreover, practitioners from the semiconductor industry who have frequently articulated their perception that academic research does not always address the real problems faced by the industry will bring in their domain knowledge to make sure that progress towards applicability and feasibility will be made during this seminar.

The Industry 4.0 vision is a frequently discussed topic in manufacturing enterprises in Europe, Asia, and North America. It is expected that advanced technologies such as Cyber-Physical Systems (CPS), Internet of Things (IoT), cloud computing, artificial intelligence, machine learning, and big data technologies enable the emergence of smart manufacturing systems. A smart factory promises to bring transparency to manufacturing facilities by integrating technological advances in computer networks, data integration, and analytics [13]. At the same time, critical questions are asked related to the benefits of Industry 4.0 ([14], [17], [15]). It is mainly criticized that the requirements and consequences of Industry 4.0 regarding future production planning and control strategies are not fully understood or not even taken into account in the overall Industry 4.0 conception, i.e., many of key decision processes are not included [14].
The semiconductor industry is capital intensive with the cost of an entire wafer fab up to nearly $10 billion US. The high cost is primarily due to extremely expensive machines, some cost up to $100 million US each. The manufacturing process is very complex due to reentrant flows in combination with very long cycle times and multiple sources of uncertainty [16]. Capacity expansions are expensive and time-consuming. The semiconductor industry is an extreme field for production planning and control solutions from an algorithmic and also from a software and information systems point of view. At the same time, the degree of automation has always been and continues to be high compared to other industries [1]. On the one hand, one can argue that some elements of smart manufacturing are already realized in wafer fabs, namely:
- most manufacturing information is available in real-time
- the manufacturing process is paperless
- lots can be uniquely identified and located
- collaborative human-machine interactions exist.
On the other hand, there are significant differences from automation efforts in manual work-intensive industries such as automotive or aircraft manufacturing where assembly operations are performed in flow lines. In addition to shop-floor control concerns, supply chain management (SCM) problems have become more and more important in the last decade in the semiconductor industry which necessitate horizontal integration of the semiconductor supply chain.
The literature with respect to an adoption of Industry 4.0 principles for semiconductor manufacturing is small and mainly on a survey or conceptual level ([6], [4], [9], [3], [10, 11]). Concrete answers towards future production planning and control strategies that exploit the new possibilities of CPSs and big data approaches to address complicated decisions involved in semiconductor supply chains are only very initially given in the literature.
The major objective of the proposed seminar was related to developing a research agenda for making smart semiconductor manufacturing decisions and the information systems to empower flexible decisions for smart production. This includes innovative modeling approaches for supply chain planning and more detailed production planning and scheduling in semiconductor manufacturing and an analysis of requirements for next-generation information systems that support such decisions. One of the expected outcomes of the seminar consisted of developing a significant draft of a concept for a simulation testbed which allows for assessing smart planning and control decisions in the semiconductor industry.
Thus, the purpose of this workshop was to bring together researchers from different disciplines including information systems, computer science, industrial engineering, supply chain management, and operations research whose central interest is in decision-making for smart semiconductor manufacturing. Practitioners from the semiconductor industry who have frequently articulated their perception that academic research does not always address the real problems faced by the industry brought in their domain knowledge to make sure that progress towards applicability and feasibility would be made during the seminar. Due to the Covid 19 pandemic, the seminar had only nine attendees from Germany who physically attended at Dagstuhl (see Section Participants). Moreover, four online talks were given by participants from US, Taiwan, and Germany. We had participants from the leading semiconductor companies Infineon Technologies and Globalfoundries.
A primary purpose of the workshop was to extend the scope of the academic research community from conventional decision-making for single wafer fabs to making smart semiconductor manufacturing decisions for entire semiconductor supply chains. The principle architecture of the planning and control system of a conventional semiconductor supply chain is shown in Figure 1.
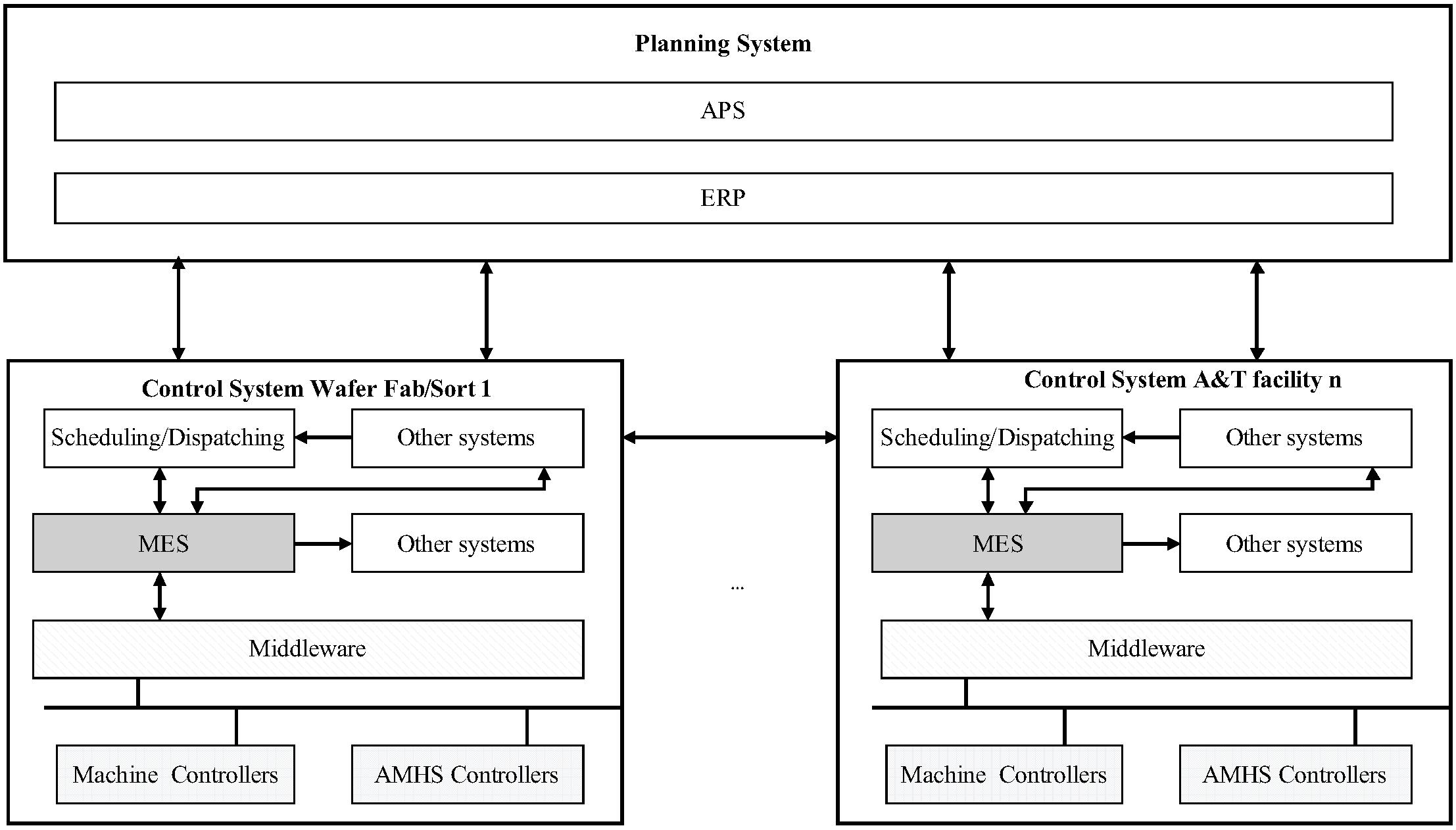
Seminar Objectives
The primary objective of the proposed seminar was to develop a research agenda for decisionmaking in smart semiconductor manufacturing. This included innovative modeling approaches for supply chain planning and detailed production planning and scheduling/dispatching in semiconductor supply chains. But it also included ideas on how to design the related future information systems.
The research agenda was developed around the following two main topics:
- Topic 1: Novel decision-making approaches that exploit the huge amount of available data and orchestrate the interrelated decisions:
- It is required to develop a better understanding of which parts of the Industry 4.0 vision are already implemented in semiconductor manufacturing and what is still missing.
- The specific automation drivers in semiconductor manufacturing compared to other industries must be identified.
- The usage of additional data which, for instance, is provided by sensors and cyberphysical systems has to be explored to make better decisions [2]. The improvement potential based on the advanced data availability must be quantified [10].
- Research is necessary to determine which decisions can or even should be integrated. Possible examples for integrated short-term decisions are job scheduling on machines and automated transportation and job scheduling and preventative maintenance planning. Integrated scheduling and process control decisions are another example. On the mid-term planning level, the integrated management of production jobs and engineering jobs is challenging. Up to 30% of all jobs in a wafer fab are engineering jobs. They compete with the production jobs for the scarce capacity of the machines. It is also interesting to make integrated production planning and inventory planning decisions in semiconductor manufacturing.
- We are interested in which changes are required (or even possible) for planning and control algorithms in smart manufacturing systems. It has to be discussed whether we expect fundamentally new algorithms or not.
- We are also interested in exploring the need for new fab layouts in the context of smart manufacturing. Initial steps towards the possible redesign of the automated material handling system (AMHS) are discussed by Ham and Kim [7] and Hwang and Jang [8].
- Dynamics and stochasticity have to be included into decision-making. Different modeling techniques that attempt to reduce the effects of stochasticity include robust optimization, approximate dynamic programming, and stochastic programming and these techniques need to be researched in the smart semiconductor manufacturing context. Different approaches to appropriately deal with stochasticity include rolling planning techniques and inventory holding strategies and these also need to be studied. Generation of scenarios and other distribution parameters for planning problems in supply chains using big data techniques have to be investigated.
- Many planning and control approaches are based on (distributed) hierarchical approaches. The role of anticipation of lower level behavior in upper level decision-making is still not well understood and has to be studied in more detail. Because many different, often autonomous decision-making entities including humans occur in semiconductor manufacturing, negotiation approaches are typical in such distributed hierarchical planning and control systems. Research is needed to investigate how such negotiation approaches can be automated and which decisions should continue to be made by humans.
- It has to be explored how to incorporate sustainability issues into decision making. For instance, taking advantage of real-time pricing in future energy markets is only reasonable when scheduling decisions can be made in real-time.
- The relationship of real-time decisions based on real-time information on the status of the shop-floor (or even the supply chain) and planning nervousness has to be studied.
- As the level of automation increases in the factory of the future, there is a need to adapt the decision-making entities to the current situation on the shop floor and at the entire supply chain level. Different machine learning paradigms have to be investigated for this purpose.
- Topic 2: Future information systems for decision support and facilitating digital transformation:
- The required changes for next-generation decision support systems have to be investigated. It is expected that decentralized decision support systems are more important than in the past.
- Alternative software solutions including software agents and service-oriented computing for planning and scheduling applications in smart semiconductor manufacturing have to be proposed.
- Alternative software solutions including software agents and service-oriented computing for planning and scheduling applications in smart semiconductor manufacturing have to be proposed.
- We have to study role of different simulation paradigms in the factory of the future/supply chain of the future.
- The benefit of digital twins in semiconductor manufacturing has to be explored. For instance, it has to be decided at what levels (e.g. factory, supply chain) they should be considered.
- Integration concepts for state-of-the-art computing techniques to get models that are computationally tractable and address the different uncertainties encountered in this industry have to be investigated for their usage in smart semiconductor manufacturing.
- Getting a better understanding the interaction of human agents with information systems in the factory of the future is required.
- Because of the complexity of semiconductor supply chains, long computing times still hinder the usage of analytic solution approaches especially for what-if analysis. The role of state-of-the-art computing techniques including parallel computing on Graphics Processing Units (GPU) machines or cloud computing techniques in decision-making for smart semiconductor manufacturing needs to be investigated.
Since the expected potential of smart manufacturing is based on advanced information and communication technologies, we think that the second topic is important and should be also addressed in the research agenda. Research related only to the first main topic is not sufficient.
Due to the inherent complexity of semiconductor supply chains, simulation of the physical supply chain is required to: a) understand the interactions between the planning and control components and the physical supply chain, b) find solution approaches to problems, and c) verify the solution approaches in the risk-free simulation environment before implementing them. Existing models do not reflect the complexity and the level of detail of current and future semiconductor supply chains. Therefore, the secondary objective of the seminar consisted in identifying the core elements of a simulation testbed which allows for assessing smart planning and control decisions in the semiconductor industry.
The Process
In the opening session, the organizers welcomed the participants. Next, the participants each introduced themselves. This was followed by an overview of the goals and objectives of the seminar and a detailed review of the seminar program including the ground rules for interactions.
The remainder of the day on Monday consisted of a keynote talk by Chen-Fu Chien and two industry overview talks (by Hans Ehm and by Steffen Kalisch). Another keynote talk was given by Leon McGinnis on Monday afternoon. The rest of Monday afternoon, Tuesday, and half a day on Wednesday were devoted to presentations and discussions about the various elements of smart decision-making in semiconductor supply chain planning and control systems as shown in Figure 1 above. There was another keynote talk delived by John Fowler on simulation modeling to reduce the impact of COVID-19. See Table 1 below for a list of topics and presenters and Section 3 for abstracts of the presentations.
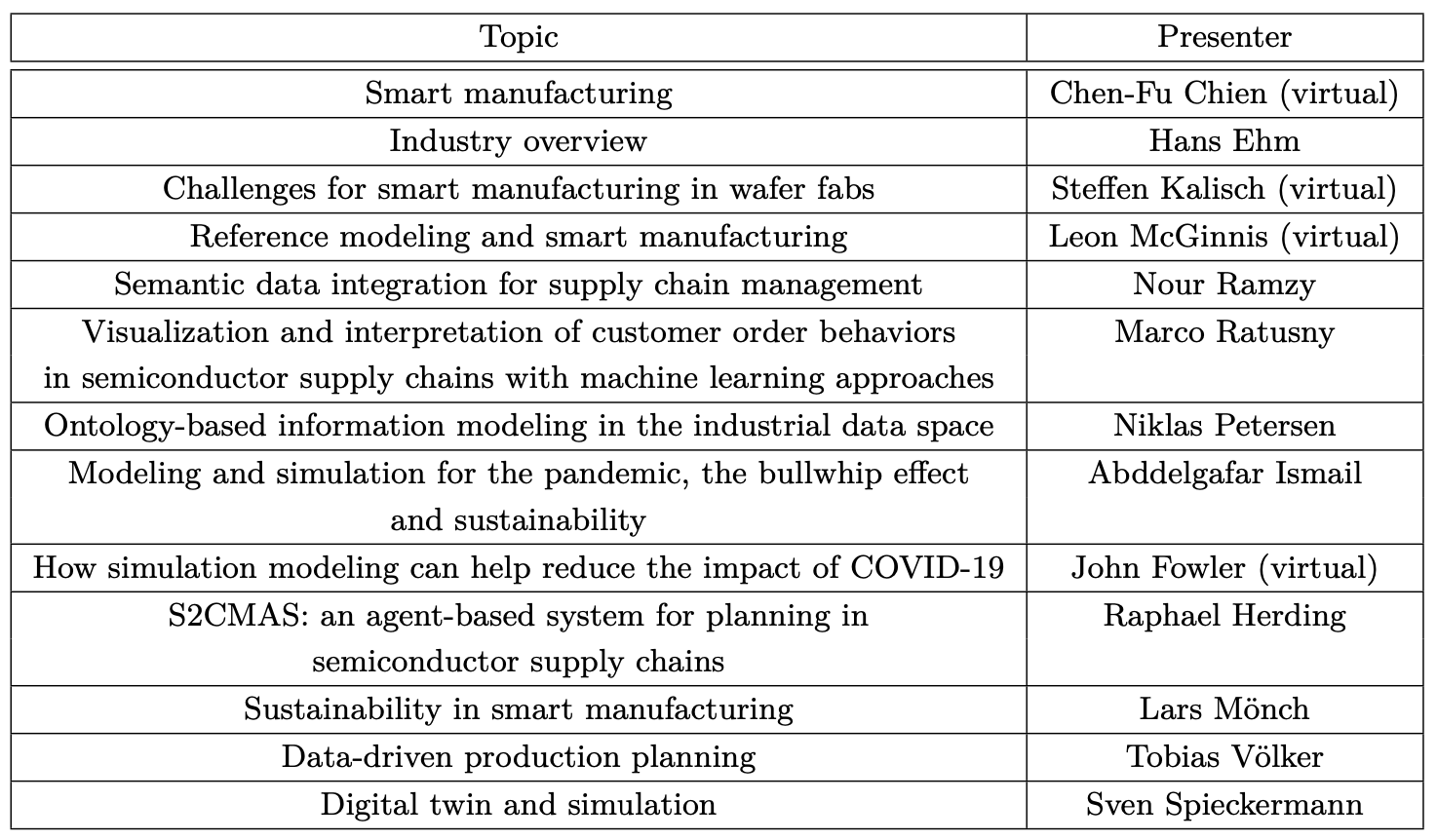
Tuesday afternoon was devoted to one breakout session with report outs on the topics in Table ef{Table2}. Section ef{breakout} has the breakout report outs. The first set of breakout sessions had two groups focus on smart planning and control decisions. The second set of breakouts on Wednesday morning again had two groups that discuss requirements for performance assessment of smart planning and control decisions in semiconductor supply chains.
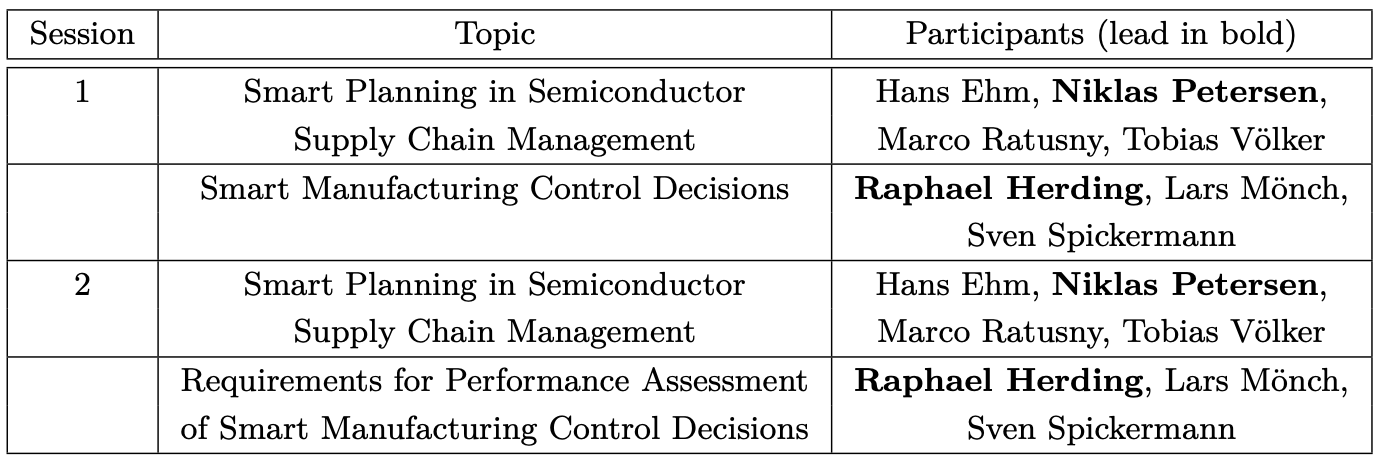
Thursday consisted of a discussion on the required core elements of a simulation testbed for assessing smart semiconductor and supply chain planning and control decisions and a wrap-up session.
Key Take Aways
There were a number of key findings and areas for future research that were identified in the seminar. We will first summarize some of the key findings and will follow this with some areas for future research.
One of the first findings was that the participants generally agreed that the term smart manufacturing is somehow fuzzy. While the different elements in Figure 1 are reasonably well understood by both the industrial and academic communities with respect to smart decision-making, the interactions between the elements are less well understood. Second, the participants generally agree that the integration of the decisions made by the different elements is often fairly ad hoc and could/should be improved. Finally, the participants generally agreed that there are new requirements for an adequate reference model for smart manufacturing in semiconductor supply chains. Although there are reasonable data sets available on the factory (i.e., the SMT2020 dataset, cf. [12]) and supply chain level (cf. [5]) no data sets exist yet that take the specifics of smart manufacturing into account.
In addition to the findings mentioned above, several areas for future research were identified. An overarching idea was that the future research should focus more on using artificial intelligence tools in smart manufacturing for semiconductor supply chains. Some of the future research areas are included below:
- Using ontologies, for instance the digital reference, for planning and control purposes.
- Developing better integration of various smart decisions made in the elements of Figure 1.
- Incorporating sustainability aspects into wafer fab and supply chain models.
- Extend existing performance assessment schemes for smart manufacturing, for instance by considering renewable energy sources such as the sun and wind.
- Extend existing simulation-based performance assessment schemes in the sense that machine learning-based planning and control schemes can be benchmarked. This requires adaptive behavior of the planning and control schemes.
Next Steps
The organizers plan to repeat the seminar after the end of the Covid 19 pandemic since several objectives of the seminar were not reached due to the limited number of participating attendees. This is especially true for the development of the research agenda for smart decisions in semiconductor manufacturing and for the simulation testbed which allows for assessing smart planning and control decisions in the semiconductor industry.
Acknowledgements
The seminar organizers would like to thank the Dagstuhl team for the provided support and the flexibility which was required due to the Covid 19 situation. The seminar also would not have been nearly as productive without the active contribution of every physical or virtual attendee, and for that the organizers are extremely grateful.
References
- Chien, C.-F., Dauzére-Pèrés, S., Ehm, H., Fowler, J. W., Jiang, Z., Krishnaswamy, S., Lee, T.-E., Mönch, L., Uzsoy, R. 2011. Modeling and Analysis of Semiconductor Manufacturing in a Shrinking World: Challenges and Successes. European Journal of Industrial Engineering, 5(3), 254-271.
- Chien, C.-F, Chuang, S.-C. 2014. A Framework for Root Cause Detection of Sub-Batch Processing System for Semiconductor Manufacturing Big Data Analytics. IEEE Transactions on Semiconductor Manufacturing, 27(4), 475-488.
- Dequeant, D., Vialletelle, P., Lemaire, P., Espinouse, M.-L. 2016. A Literature Review on Variability in Semiconductor Manufacturing: The Next Forward Leap to Industry 4.0. Proceedings of the 2016 Winter Simulation Conference, 2598-2609.
- Ehm, H., Mönch, L. 2016. Von der Digitalen Fabrik zur Digitalen Lieferkette in der Halbleiterindustrie: Bestandsaufnahme, Lösungsansätze und viele Herausforderungen. Obermaier, R. (ed.) Industrie 4.0 als unternehmerische Gestaltungsaufgabe, Springer/ Gabler, 149-158.
- Ewen, H., Mönch, L., Ehm, H., Ponsignon, T., Fowler, J. W., Forstner, L. 2017. A Testbed for Simulating Semiconductor Supply Chains. IEEE Transactions on Semiconductor Manufacturing, 30(3), 293-305.
- Forstner, L., Dümmler, M. 2014. Integrierte Wertschöpfungsnetzwerke – Chancen und Potenziale durch Industrie 4.0. Elektrotechnik und Informationstechnik, 131(7), 199-201.
- Ham, A., Kim, D. J. 2017. Scheduling of Drone-based Material Transfer System in Semiconductor Manufacturing. Proceedings of the 2017 Winter Simulation Conference, 3726-3732.
- Hwang, I., Jang, Y. J. 2020. Q( ) Learning-based Dynamic Route Guidance Algorithm for Overhead Hoist Transport systems in Semiconductor Fabs. International Journal of Production Research, 58(4), 1199-1221.
- Kaufmann, T., Forstner, L. 2017. Die horizontale Integration der Wertschöpfungskette in der Halbleiterindustrie – Chancen und Herausforderungen. T. Bauernhansl, M. ten Hompel, B. Vogel-Heuser (eds.) Industrie 4.0 in Produktion, Automatisierung und Logistik, Wiesbaden, 359-367.
- Khakifirooz, M., Fathi, M., Wu, K. 2019a. Development of Smart Semiconductor Manufacturing: Operations Research and Data Science Perspectives, IEEE Access, 7.
- Khakifirooz, M., Fathi, M., Chien, C.-F., Pardalos, P. M. 2019b. Management Suggestions for Process Control of Semiconductor Manufacturing: An Operations Research and Data Science Perspective. Blondin, M. J., Pardalos, P. M., Sáez, J. S. (eds.) Computational Intelligence and Optimization Methods for Control Engineering, Springer, 245-274.
- Kopp, D., Hassoun, M., Kalir, A., Mönch, L. 2020. SMT2020 – A Semiconductor Manufacturing Testbed. IEEE Transactions on Semiconductor Manufacturung, 33(4), 522 – 531.
- Lee, J. 2015. Smart Factory Systems. Informatik-Spektrum, 38(3), 230-235.
- Mertens, P. 2014. Industrie 4.0 = CIM 2.0? Industrie Management, 30(1), 27-30.
- Mertens, P., Barbian, D. 2019. Industrie 4.0 und Integrierte Informationsverarbeitung. Obermaier, R. (ed.) Handbuch Industrie 4.0 und Digitale Transformation, Springer/Gabler, 47-71.
- Mönch, L., Fowler, J. W., Mason, S. J. 2013. Production Planning and Control for Semiconductor Wafer Fabrication Facilities. New York: Springer.
- Sucky, E., Gampl, M., Ruh, A., Stelzer, N., Weidinger, J. 2018. Industrie 4.0: Marketingkampagne oder Revolutionsbeginn? https://opus4.kobv.de/opus4-bamberg/frontdoor/index/index/docId/46573.

- Hans Ehm (Infineon Technologies - München, DE) [dblp]
- Raphael Herding (Westfälische Hochschule - Bocholt, DE) [dblp]
- Abdelgafar Ismail (Infineon Technologies - München, DE)
- Lars Mönch (FernUniversität in Hagen, DE) [dblp]
- Niklas Petersen (eccenca GmbH - Leipzig, DE) [dblp]
- Nour Ramzy (Infineon Technologies - München, DE) [dblp]
- Marco Ratusny (Infineon Technologies - München, DE)
- Sven Spieckermann (SimPlan AG - Hanau, DE) [dblp]
- Tobias Völker (FernUniversität in Hagen, DE)
Related Seminars
Classification
- Multiagent Systems
- Other Computer Science
- Systems and Control
Keywords
- Modeling
- Simulation
- Smart Manufacturing
- Analytics
- Semiconductor Manufacturing