Dagstuhl Seminar 20372
Beyond Adaptation: Understanding Distributional Changes
( Sep 06 – Sep 11, 2020 )
Permalink
Organizers
- Niall Adams (Imperial College London, GB)
- Vera Hofer (Universität Graz, AT)
- Eyke Hüllermeier (Universität Paderborn, DE)
- Georg Krempl (Utrecht University, NL)
- Geoffrey Webb (Monash University - Clayton, AU)
Contact
- Michael Gerke (for scientific matters)
- Annette Beyer (for administrative matters)
Schedule
The world is dynamically changing and non-stationary. This is reflected by the variety of methods that have been developed in statistics, machine learning, and data mining to detect these changes, and to adapt to them. Nevertheless, most of this research views the changing environment as a black box data generator, to which models are adapted (Figure 1, left).
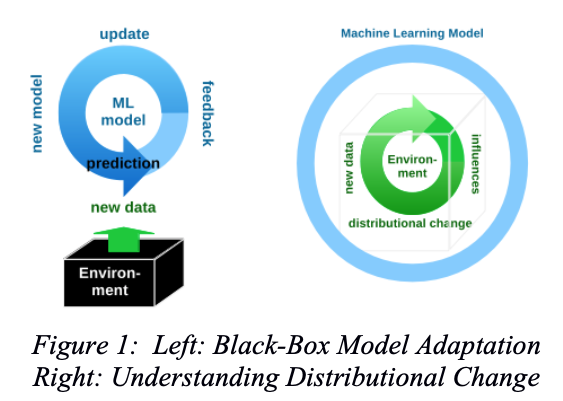
This Dagstuhl Seminar takes a change mining point of view, by focusing on change as a research subject of its own. This aims to make the distributional change process in the data generating environment transparent (Figure 1, right). It seeks to establish a better understanding of the causes, nature and consequences of distributional changes. Thereby, it aims to address the following research questions:
- Understanding which scenarios and types of change are relevant in practical applications
- How to model such types of change effectively
- How to detect, verify, and measure types of change
- How to establish bounds for distributional change, or for predictive performance under change
- How to effectively adapt prediction models to the different types of change
- How to visualise change, and how to highlight individual types of change
- How to evaluate techniques for the above questions
Thereby, this seminar will bridge communities where in separate lines of research some parts of these questions are already studied. These include data stream mining, where focus is on concept drift detection and adaptation, transfer learning and domain adaptation in machine learning and algorithmic learning theory, change point detection in statistics, adversarial generators in adversarial machine learning, and the evolving and adaptive systems community. Therefore, this seminar aims to bring together researchers and practitioners from these different areas, and to stimulate research towards a thorough understanding of distributional changes.

The world is dynamically changing and non-stationary. This is reflected by the variety of methods that have been developed to detect changes and adapt to them. These contributions originate from various communities, including statistics, machine learning, data mining, and the evolving and adaptive systems community. Nevertheless, most of this research views the changing environment as a black-box data generator, to which models are adapted (Fig. 1).
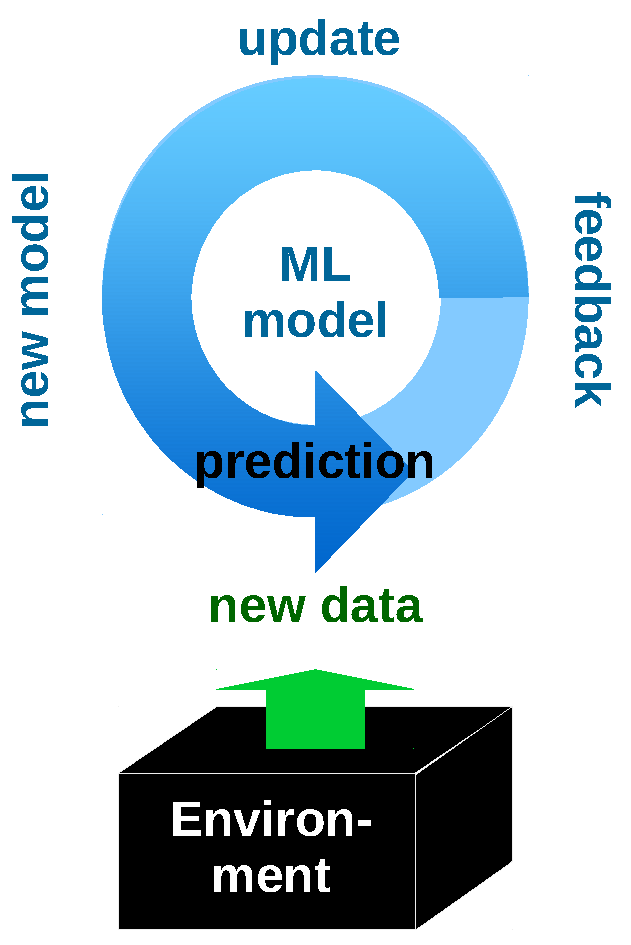
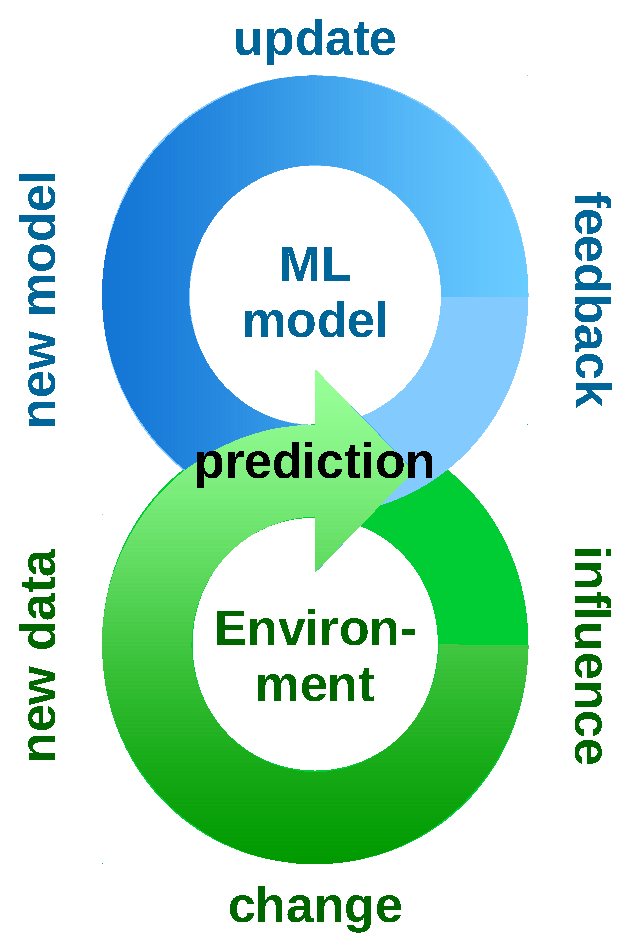
The aim of this seminar was to put the focus on the distributional change itself, i.e., to make the process itself more transparent and a subject of research in its own right (Fig. 2). In its endeavour to understand causes, nature and consequences of distributional change, the seminar brought together researchers from communities in which related questions have already been studied, albeit in separate lines of research. These include data stream mining, time series and sequence analysis, domain adaptation and transfer learning, subgroup discovery and emerging pattern mining.
Data stream mining studies data that arrives either one-by-one or in batches over time, and where the data generating process is often non-stationary. This requires computationally efficient approaches that are capable to detect and adapt to distributional changes. In this literature, the latter are commonly denoted as concept drift, population drift, or shift. Related to this seminar are in particular the problems of identifying change or irregularities in data streams, such as outlier detection [1], anomaly detection [2], change detection [3], change diagnosis [4], change mining [5], drift mining [6], and drift understanding [7].
Time series analysis studies data observed over a time course typically exhibiting some time dependencies. The correspondence of distributional change in this literature are distributional structural breaks or change points. Thus, of particular interest are the problems of statistical change point analysis, see e.g. the books by [8, 9, 10] or recent survey articles [12, 13]. A different line of research focuses on smallest detection delay for changes in sequentially observed data, see e.g. the recent books by [14, 15]. Of recent interest are also methods for the localization of multiple change points also known as data segmentation methodology, see e.g. the recent survey articles [16, 17, 18, 19, 20]. Of further interests is an early classification of time series [21].
Domain adaptation and transfer learning study the problem of transferring knowledge between domains or tasks. While there is not necessarily a temporal relationship between domains or tasks, distributional differences between domains are studied under the notion of dataset shift. Related problems of particular interest are lifelong learning [22] and unsupervised domain adaptation [23].
Subgroup discovery studies the problem of finding subgroups that show an unusual distribution for a target variable. There is not necessarily a temporal relationship between subgroup. Of particular interest is exceptional model mining, which studies the problem of finding subgroups, where a model fitted to that subgroup is somehow exceptional [24]. Another related area is emerging pattern mining [25] for identifying emerging trends in time-stamped databases.
Topics Discussed in the Seminar
The seminar identified several key research questions around understanding distributional changes:
- Understanding the practical relevance of different scenarios and types of change.
- How to model such types of change effectively.
- How to detect, verify, and measure types of change.
- How to effectively adapt prediction models to the different types of change.
- How to establish bounds for distributional change, or for predictive performance under change.
- How to visualise change, and how to highlight individual types of change.(interactively).
- How to evaluate techniques for the above questions.
Due to the limited time, discussion has focused mostly on the first four research questions, with plans to address the remaining questions in a follow-up seminar.
Program Overview
This one-week seminar was structured such that plenary sessions formed a frame around parallel break-out group sessions. It was opened with plenary sessions on Monday and Tuesday morning, where four tutorial served to establish a common vocabulary and understanding between the participants from the different communities. In the subsequent four half-days, 13 spotlight talks were organised, each followed by discussions in break-out groups, and each closing by a short bring-back plenary session. The seminar closed by two plenary sessions on Friday morning, where action plans for further steps on research and collaboration were discussed.
Outcomes
As detailed in the description of the sessions below, and in particular for the plenary session, differences in the terminology, concepts and common assumptions used in the different communities were identified as an important challenge towards common understanding of distributional changes. Therefore, a potential follow-up collaboration will focus on a joint publication that provides a mapping of terms and concepts. In particular, it should work out the notion of change (and representation) in data streams and time series, as well as in domain adaptation with multiple temporally connected source domains.
References
- Shiblee Sadik and Le Gruenwald. Research issues in outlier detection for data streams. ACM SIGKDD Explorations Newsletter, 15(1):33–40, 2014.
- Varun Chandola, Arindam Banerjee, and Vipin Kumar. Anomaly detection: A survey. ACM computing surveys (CSUR), 41(3):15, 2009.
- Dang-Hoan Tran, Mohamed Medhat Gaber, and Kai-Uwe Sattler. Change detection in streaming data in the era of big data: models and issues. ACM SIGKDD Explorations Newsletter, 16(1):30–38, 2014.
- Charu C. Aggarwal. On change diagnosis in evolving data streams. IEEE Transactions on Knowledge and Data Engineering, 17(5):587–600, 2005.
- Mirko Böttcher, Frank Höppner, and Myra Spiliopoulou. On exploiting the power of time in data mining. ACM SIGKDD Explorations Newsletter, 10(2):3–11, 2008.
- Vera Hofer and Georg Krempl. Drift mining in data: A framework for addressing drift in classification. Computational Statistics and Data Analysis, 57(1):377–391, 2013.
- Geoffrey Webb, Loong Kuan Lee, Bart Goethals, and Francois Petitjean. Analyzing concept drift and shift from sample data. Data Mining and Knowledge Discovery, 32(5):1179–1199, 2018.
- Miklós Csörgö and Lajos Horváth. Limit Theorems in Change-point Analysis, volume 18. John Wiley & Sons Inc, 1997.
- Jie Chen and Arjun K Gupta. Parametric statistical change point analysis: with applications to genetics, medicine, and finance. Springer Science & Business Media, 2nd edition, 2011.
- B. E. Brodsky and B. S. Darkhovsky. Nonparametric Methods in Change-point Problems. Springer, 1993.
- John AD Aston and Claudia Kirch. Detecting and estimating changes in dependent functional data.Journal of Multivariate Analysis, 109:204–220, 2012
- Alexander Aue and Lajos Horváth. Structural breaks in time series. Journal of Time Series Analysis, 34:1–16, 2013.
- Lajos Horváth and Gregory Rice. Extensions of some classical methods in change point analysis. TEST, 23:1–37, 2014.
- Alexander Tartakovsky, Igor Nikiforov, and Michele Basseville. Sequential analysis: Hypothesis testing and changepoint detection. CRC Press, 2014.
- Alexander Tartakovsky. Sequential Change Detection and Hypothesis Testing: General Non-iid Stochastic Models and Asymptotically Optimal Rules. CRC Press, 2019.
- Idris A Eckley, Paul Fearnhead, and Rebecca Killick. Analysis of changepoint models. In David Barber, A. Taylan Cemgil, and Silvia Chiappa, editors, Bayesian Time Series Models, chapter 10, pages 205–224. Cambridge University Press, Cambridge, 2011.
- Samaneh Aminikhanghahi and Diane J Cook. A survey of methods for time series change point detection. Knowledge and Information Systems, 51:339–367, 2017.
- Haeran Cho and Claudia Kirch. Data segmentation algorithms: Univariate mean change and beyond. arXiv preprint arXiv:2012.12814, 2020.
- Paul Fearnhead and Guillem Rigaill. Relating and comparing methods for detecting changes in mean. Stat, page e291, 2020.
- Charles Truong, Laurent Oudre, and Nicolas Vayatis. Selective review of offline change point detection methods. Signal Processing, 167:107299, 2020.
- Zhengzheng Xing, Jian Pei, and Eamonn Keogh. A brief survey on sequence classification. ACM Sigkdd Explorations Newsletter, 12(1):40–48, 2010.
- Anastasia Pentina and Ruth Urner. Lifelong learning with weighted majority votes. In Neural Information Processing Systems, volume 29, pages 3619–3627, 2016.
- Shai Ben-David and Ruth Urner. On the hardness of domain adaptation and the utility of unlabeled target samples. In International Conference on Algorithmic Learning Theory, pages 139–153, 10 2012.
- Wouter Duivesteijn and Julia Thaele. Understanding where your classifier does (not) work. In Albert Bifet, Michael May, Bianca Zadrozny, Ricard Gavalda, Dino Pedreschi, Francesco Bonchi, Jaime Cardoso, and Myra Spiliopoulou, editors, Machine Learning and Knowledge Discovery in Databases, pages 250–253, Cham, 2015. Springer International Publishing.
- Petra Kralj Novak, Nada Lavrac, and Geoffrey I Webb. Supervised descriptive rule discovery: A unifying survey of contrast set, emerging pattern and subgroup mining. Journal of Machine Learning Research, 10(2), 2009.

- Barbara Hammer (Universität Bielefeld, DE) [dblp]
- Claudia Kirch (Universität Magdeburg, DE) [dblp]
- Georg Krempl (Utrecht University, NL) [dblp]
- Mykola Pechenizkiy (TU Eindhoven, NL) [dblp]
- Sarah Schnackenberg (Köln, DE)
- Myra Spiliopoulou (Universität Magdeburg, DE) [dblp]
- Dirk Tasche (FINMA - Bern, CH)
- Andreas Theissler (Hochschule Aalen, DE)
- Amir Abolfazli (Leibniz Universität Hannover, DE)
- Shai Ben-David (University of Waterloo, CA) [dblp]
- Antoine Cornuéjols (AgroParisTech - Paris, FR) [dblp]
- Saso Dzeroski (Jozef Stefan Institute - Ljubljana, SI) [dblp]
- Johannes Fürnkranz (Johannes Kepler Universität Linz, AT) [dblp]
- Joao Gama (INESC TEC - Porto, PT) [dblp]
- Gerhard Gößler (Universität Graz, AT)
- Vera Hofer (Universität Graz, AT) [dblp]
- Eyke Hüllermeier (Universität Paderborn, DE) [dblp]
- Yun Sing Koh (University of Auckland, NZ)
- Mark Last (Ben Gurion University - Beer Sheva, IL)
- Loong Kuan Lee (Monash University - Caulfield, AU)
- Pavlo Mozharovskyi (Telecom Paris, FR)
- Eirini Ntoutsi (Leibniz Universität Hannover, DE) [dblp]
- Arno Siebes (Utrecht University, NL) [dblp]
- Jurek Stefanowski (Poznan University of Technology, PL)
- Ruth Urner (York University - Toronto, CA) [dblp]
- Geoffrey Webb (Monash University - Clayton, AU) [dblp]
- Indre Žliobaite (University of Helsinki, FI)
Classification
- artificial intelligence / robotics
Keywords
- statistical machine learning
- data streams
- concept drift
- non-stationary non-iid data
- change mining